有效使用统计数据的十个简单规则
2017-03-21 MedSci MedSci原创
为此,一个统计学家组成的团队,其中包括卡内基梅隆大学的罗伯特E.卡斯,撰写了论文“有效统计实践的十个简单规则”,并发表在PLOS计算生物学期刊的“十个简单规则”系列,目的是帮助研究团体,特别是那些不是统计专家的科学家们,或缺乏专门统计人员的科研团队,了解如何避免统计陷阱,进行不合理的统计推理。 “我们作为研究人员,中心工作和日常任务就是和数据进行沟通,针对我们想要解决的问题,破译其中数据
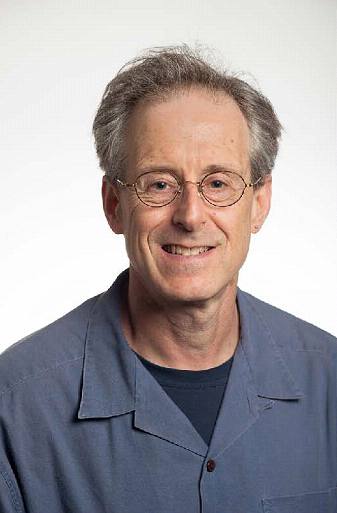
“我们作为研究人员,中心工作和日常任务就是和数据进行沟通,针对我们想要解决的问题,破译其中数据所蕴含的意义,” 统计学和机器学**教授卡斯写道,他也是认知神经基础中心代理主任。
他继续说,“即使没有正确的语言命令,基本的对话也能实现(可以按照常规进行),但是在解决许多微妙问题,确保解读过程中没有丢失关键信息,以及增加你的研究结果经得起时间考验的可能性方面,原则性的统计分析是至关重要的。”
这些原则是2016年6月9日在网上发布的,已经收到了非常多的关注,到目前为止,已经有超过37000页的评论,使它成为这个系列中,最受关注的前20篇论文之一,论文总数是60篇。对于他们受关注的程度,卡内基梅隆大学心理系主任Michael J. Tarr一点也不惊讶。
“科学,特别是心理学和神经科学的领域,在最近几年里已经受到越来越多的严格审查,因为统计方法使用不当”Tarr说。“Kass和他的同事们提出的简单易懂的指南,将**地提醒学生和教师,统计对于基础研究的重要性。他们的论文是针对那些对科学研究认真严谨的人来说,是非常及时的,而且是必读之作。”
十条规则包括:
① 统计方法应使数据回答的科学问题
在调查的早期,与统计人员合作往往是最有帮助的,因为不专业的统计人员往往专注于使用何种技术来分析数据,而不是考虑数据回答基本科学问题的所有方式。
② 数据总有误差和缺失
变量有很多形式,为了表示不确定性,了解原始数据的优劣非常关键。这有助于确定系统误差来源。
③ 事前规划非常重要
在数据分析的最初阶段,明确分析的目的,这会让你在数据分析阶段不至于抓狂。另外,精心组织的数据收集工作,也能**简化分析过程,使其更加严谨。
④ 关注数据质量
说到数据分析,使用的数据如果不准确,会导致“垃圾数据得出垃圾结论”的结果。现代数据收集的复杂性需要许多技术功能方面的假设,包括数据预处理技术,这些技术可以产生重要影响,也很容易被忽视。
⑤ 统计分析不仅仅是一系列的计算
统计软件是辅助分析的工具,而不是定义它们的方法。科学语境非常关键,统计分析的关键是把分析方法与科学问题紧密联系在一起。
⑥ 保持简单
简单胜过复杂。大量的数据测量,解释变量之间的相互作用,非线性机制的作用,丢失的数据,混杂,采样偏差和其他因素,都需要增加模型的复杂性。
但是,要记住,设计好数据分析框架,并按照计划实施,往往可以让分析方法变得简单,并得出正确的结果。
⑦ 提供变量的评估
统计分析的一个基本目的是帮助评估不确定性,一般是以标准差或置信区间的形式,统计建模和推断最成功之处在于,它可以确定数据样本的标准差估计。报告结果时,提供统计误差的结果非常重要。
⑧ 检查你的假设
广泛使用的统计软件使数据分析变得容易,你无须关注固有的假设,这会导致不精确或错误结果的风险。因此,了解分析方法中固有的假设,尽可能去理解和评估这些假设至关重要。
⑨ 如果可能的话,对结果进行复制
在理想情况下,复制是由独立的调查人员承担的。经得起时间考验的科学成果能够经得起各种不同的,但是密切相关的情况去验证。在许多情况下,完整的复制非常困难或者不可能,比如大规模的临床试验结果。在这种情况下,最低标准是遵循规则10。
⑩ 使你的分析结论可复制
给定相同的数据集,连同一个完整的分析描述,就应该可以复制你的数据表,数字和统计推断。在分析过程中,通过系统分析步骤,通过共享用于产生结果的数据和代码,并遵照公认的统计规则,能够显著提高复制分析结论的能力。
“在统计学领域,我对确定统计规则的价值深信不疑,并把它们清楚和简洁地表达出来,”Kass说。“这10个简单规则系列非常棒,已经证明了它作为科学概念分析框架的价值。这篇文章需要辛苦的工作,但我们有一个伟大的团队,我对结果非常满意。”
A summary of the 10 rules:
#1 – Statistical Methods Should Enable Data to Answer Scientific Questions
Collaborating with statisticians is often most helpful early in an investigation because inexperienced users of statistics often focus on which technique to use to analyze data, rather than considering all of the ways the data may answer the underlying scientific question.
#2 – Signals Always Come With Noise
Variability comes in many forms, but it is crucial to understand when it is good and when it is noise in order to express uncertainty. It also helps to identify likely sources of systematic error.
#3 – Plan Ahead, Really Ahead
Asking questions at the design stage can save headaches at the analysis stage. Careful data collection also can greatly simplify analysis and make it more rigorous.
#4 – Worry About Data Quality
When it comes to data analysis, “garbage in produces garbage out.” The complexity of modern data collection requires many assumptions about the function of technology, often including data pre-processing technology, which can have profound effects that can easily go unnoticed.
#5 – Statistical Analysis Is More Than a Set of Computations
Statistical software provides tools to assist analysis, not define them. The scientific context is critical, and the key to principled statistical analysis is to bring analytical methods into close correspondence with scientific questions.
#6 – Keep it Simple
Simplicity trumps complexity. Large numbers of measurements, interactions among explanatory variables, nonlinear mechanisms of action, missing data, confounding, sampling biases and other factors can require an increase in model complexity. But, keep in mind that a good design, implemented well, can often allow simple methods of analysis to produce strong results.
#7 – Provide Assessments of Variability
A basic purpose of statistical analysis is to help assess uncertainty, often in the form of a standard error or confidence interval, and one of the great successes of statistical modeling and inference is that it can provide estimates of standard errors from the same data that produce estimates of the quantity of interest. When reporting results, it is essential to supply some notion of statistical uncertainty.
#8 – Check Your Assumptions
Widely available statistical software makes it easy to perform analyses without careful attention to inherent assumptions, and this risks inaccurate, or even misleading, results. It is therefore important to understand the assumptions embodied in the methods and to do whatever possible to understand and assess those assumptions.
#9 – When Possible, Replicate!
Ideally, replication is performed by an independent investigator. The scientific results that stand the test of time are those that get confirmed across a variety of different, but closely related, situations. In many contexts, complete replication is very difficult or impossible, as in large-scale experiments such as multi-center clinical trials. In those cases, a minimum standard would be to follow Rule 10.
#10 – Make Your Analysis Reproducible
Given the same set of data, together with a complete description of the analysis, it should be possible to reproduce the tables, figures and statistical inferences. Dramatically improve the ability to reproduce findings by being very systematic about the steps in the analysis, by sharing the data and code used to produce the results and by following accepted statistics best practices.
In addition to Kass, the co-authors are Johns Hopkins University’s Brian S. Caffo, North Caroline State University’s Marie Davidian, Harvard University’s Xiao-Li Meng, Bin Yu of the University of California Berkeley, and Nancy Reid of the University of Toronto.
“I am a big believer in the value of identifying major ideas in statistics, and stating them clearly and concisely,” Kass said. “The 10 simple rules series is terrific, having proven its worth as a format for high-level scientific concepts. This article was pretty hard work, but we had a great team and I was extremely happy with the result.”
本网站所有内容来源注明为“梅斯医学”或“MedSci原创”的文字、图片和音视频资料,版权均属于梅斯医学所有。非经授权,任何媒体、网站或个人不得转载,授权转载时须注明来源为“梅斯医学”。其它来源的文章系转载文章,或“梅斯号”自媒体发布的文章,仅系出于传递更多信息之目的,本站仅负责审核内容合规,其内容不代表本站立场,本站不负责内容的准确性和版权。如果存在侵权、或不希望被转载的媒体或个人可与我们联系,我们将立即进行删除处理。
在此留言
学习了,以后在工作中会注意的
78
值得学习谢谢共享
70
临床医生做研究最好是与统计专家组成团队。
0